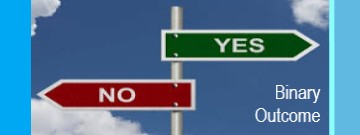
A Binary Outcome implies there are only two possible outcomes to a given situation. For example, in merger arbitrage, the deal will consummate successfully or it will fail. Despite there being a potential revision to the original terms of the deal, ultimately, the deal either fails or closes.
In statistics, binary data is categorical data that can take either one of two values which cannot be compared numerically. The binary outcome is a general term for data that can be counted however by using a series of “ones” and “zeros” to represent success or failure of each individual trial or event.
Event | Positive Outcome | Negative Outcome |
---|---|---|
merger or acquisition | success | failure |
response to a closed-end question | yes | no |
presence or absence of some feature | present | not present |
truth or falsehood of a statement | true | false |
Binary Outcome Usage
Binary outcomes have applications in many fields beyond statistics. Computer science assigns a binary outcome to a “bit” where the value is either 0 or 1. This “bit” is then combined with additional “bits” to make a larger data string. Statistical and mathematical applications also make great use of binary outcomes.
It is from the field of mathematics that the concept of a binary outcome can be applied to finance and investing. Situations resulting in two potential payoffs, such as mergers & acquisitions end with either a positive or negative result. Options trading is another example of an investment strategy with binary outcomes. In this instance, taking a position in either calls and puts will ultimately lead to those options either expiring in the money (ITM) or expiring out the money (OTM). In other words, they will have or they will not.
When calculating the Deal Closing Probability (DCP) traders may elect to use a logistic regression can be used to model a binary response. The deal outcome is binary and has only two outcomes. By applying a logistic regression, it allows the trader to estimate the probability of the binary outcome based on the values of the explanatory variables. With this figure, traders are then able to more accurately assess the profitability of a merger arbitrage investment. For more on this topic see Applied Logistic Regression by David W. Hosmer Jr., Stanley Lemeshow & Rodney X. Sturdivant.
Well known variable that have previously been highlighted in academic literature as reliable indicators of deal success are target management attitude, relative firm size, payment type (such as cash deal or stock swap) and industry amongst others. When added to a logistic regression the outcome is prevented from going above 100% which may happen in a standard regression model.